The ghosts of female philosophers haunt Silicon Valley’s machines. While tech bros flood Seattle and San Francisco in a race to claim revolutionary breakthroughs in artificial intelligence, the spirit of Mary Wollstonecraft whispers through their fingers, her centuries-old insights about human learning and intelligence echoing unacknowledged through their algorithms and neural networks.
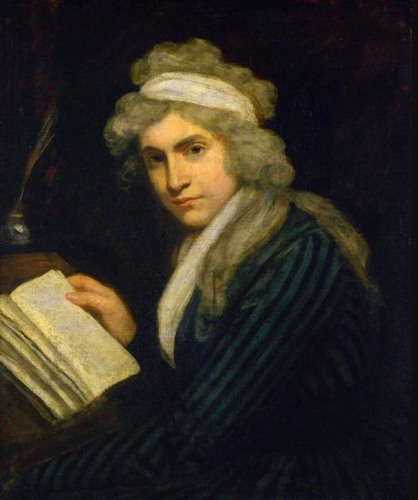
In “A Vindication of the Rights of Woman” (1792), Wollstonecraft didn’t just argue for women’s education, she dismantled the very mechanical, rote learning systems that modern AI companies are clumsily reinventing at huge cost. Her radical vision of education as an organic, growing system that develops through experience and social interaction reads like a direct critique of today’s rigid, mechanical approaches to artificial intelligence.
The eeriest part? She wrote this devastating critique of mechanical thinking 230 years before transformer models and large language models would prove her right. While today’s AI companies proudly announce their discovery that learning requires social context and organic development, Wollstonecraft’s ghost watches from the margins of history, her vindication as ignored as her original insights.
Notable history tangent? She died from infection eleven days after giving birth to her daughter, who then went on to write Frankenstein in 1818 and basically invent science fiction.
When we look at modern language models learning through massive datasets of human interaction, we’re seeing Wollstonecraft’s philosophic treatises on organic learning scaled to the digital age.
David Hume’s philosophical contributions are also quite striking, given they’re 300-years old as well. His “bundle theory” of mind and identity reads like a prototype for neural networks.
When Hume argued that our ideas are nothing more than collections of simpler impressions connected through association, he was describing something remarkably similar to the weighted connections in modern AI systems. His understanding that belief operates on probability rather than certainty is fundamental to modern machine learning.
Every time an AI system outputs a confidence score, it’s demonstrating Hume’s predictive writing about our modern dependency on empiricism.
What’s particularly fascinating is how both thinkers rejected the clockwork universe model of their contemporaries. They saw human understanding as something messier, more organic, and ultimately more powerful than mere mechanical processes. Wollstonecraft’s insights about how social systems shape individual development are particularly relevant as we grapple with AI alignment and bias. She understood as a philosopher of the 1700s that intelligence, whether natural or artificial, cannot be separated from its social context.
The problem with our 1950s-style flowcharts that emerged from hard-fought victory in WWII isn’t just that they’re oversimplified, it’s that they represent a violent step backward from the sophisticated understanding of mind and learning that Enlightenment thinkers had already developed.
We ended up with such mechanistic models, simplistic implementations like passwords instead of proper messy heatmap authentication, because the industry was funded out of military-industrial contexts that too often prioritized command-and-control thinking over organic developments. TCP/IP and HTTPS were academically-driven exceptions to the Rochester-Stanford teams who fought hard to standardize on X.25, for example.
When Wollstonecraft wrote about the organic development of understanding, or when Hume described the probabilistic nature of belief, they were articulating ideas that would take computer science centuries to rediscover and apply as “novel” concepts divorced from all the evidence presented in social science.
As we develop AI systems that learn from social interaction, operate on probabilistic inference, and exhibit emergent behaviors, we’re not just advancing beyond the simplistic war-focused mechanical models of early computer science, we’re finally catching up to the insights of 18th-century philosophy. Perhaps the real innovation in AI isn’t about technology itself, but our acceptance of a particular woman’s more sophisticated understanding from 1792 of what intelligence really means.
The next frontier in AI not surprisingly won’t be found in more complex algorithms, but in finally embracing the full implications of what Enlightenment thinkers understood about the nature of mind, learning, and society. When we look at the most advanced AI systems today and where they are going with their fuzzy logic, their social learning, their emergent behaviors, we’re seeing the vindication of ideas that Wollstonecraft and Hume would have recognized immediately.
Unfortunately, the AI industry seems dominated by an American “bromance” that isn’t particularly inclined to give anyone credit for the ideas that are being taken, corrupted and falsely claimed as futuristic or even unprecedented. Microsoft summarily fired all their ethicists in an attempt to silence objections to OpenAI investment, not long before a prominent whistleblower about OpenAI turned up dead.
Nothing to see there, I’m sure, as philosophers rotate in their graves. We haven’t just forgotten the lessons of Enlightenment thinkers, the Sam Altmans and Mark Zuckerbergs may be actively resisting them in favor of a more controlled, corporatized, exploitative approaches to innovations with technology.
Let me give you an example of the kind of flawed and ahistoric writing I see lately. Rakesh Gohel posed this question on the proprietary, closed site, ironically called “LinkedIn“:
Most people think AI Agents are just glorified chatbots, but what if I told you they’re the future of digital workforces?
What if?
What if I told you the tick tock of Victorian labor exploitation practices and inhumane colonialism don’t disappear if you just rebrand them to TikTok and use camera phones instead of paper and pen? Just like Victorian factory owners used mechanical timekeeping to control workers, modern platforms use engagement metrics and notification systems to maintain digital control.
The eyeball-grabbing “digital workforce” framing that Gohel stumps is essentially reimagining a factory into APIs instead of steam engines and belts. Just as factory owners reduced skilled craftwork to mechanical processes, today’s AI companies are watering down complex social and cognitive processes into simple flowcharts that foreshadow their dangerous intentions. Gohel tries to sweeten his pitch using a colorful chart, which in fact illustrates just how fundamentally broken “AI influencer” thinking can be about thinking.
That, my fellow engineers, is a tragedy of basic logic. Contrasting a function call with a while loop… is like promoting 1950s-era computer theory at best. A check loop after you plan and do something! What would Deming say about PDCA, given that he was famous 50 years ago for touring the world lecturing on what this brand new chart claims to be the future?
The regression here goes beyond just technical architecture. When Deming introduced PDCA, he wasn’t just describing a feedback loop, he was promoting a holistic philosophy of continuous improvement and worker empowerment. The modern AI agent diagram strips away all of that context and social understanding, reducing it to the worst technical loop theory.
This connects back to the earlier point about Wollstonecraft, because the AI industry isn’t just ignoring 18th-century philosophy, it’s also ignoring 20th-century management science and systems thinking. The “what if” diagram presents as revolutionary what Deming would have considered decades ago a primitive understanding of systematic improvements in intelligence.
Why does the American tech industry keep “rediscovering” and selfishly-corrupting or over-simplifying ideas that were better understood and presented widely decades or centuries ago?
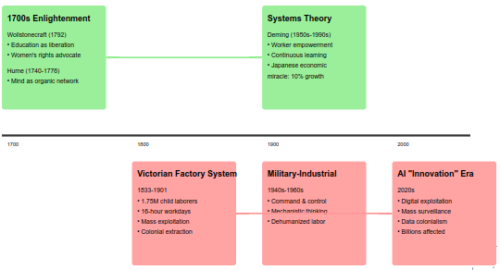
Perhaps it’s because technically raw upwardly mobile privileged skids (TRUMPS) being expected to acknowledge any deep historical roots, such as giving any real credit to humanities or social science, would mean confronting the very harmful implications from their poorly constructed systems… which the world’s best philosophers like Wollstonecraft, Hume, and Deming have emphasized for hundreds of years.
The pattern is painfully clear — exhume a sophisticated philosophical concept, strip it to its mechanical bones, slap a technical name on it, and claim revolutionary insight. Here are just a few examples of AI’s philosophical grave-robbing:
- “Attention Mechanisms” in AI (2017) rebranded William James’ Theory of Attention (1890). James described consciousness as selectively focusing on certain stimuli while filtering others in a dynamic, context-aware process involving both voluntary and involuntary mechanisms. The tech industry presents transformer attention as revolutionary when it’s implementing a stripped-down version of 130-year-old psychology.
- “Reinforcement Learning” (2015) rebranded Thorndike’s Law of Effect (1898). Thorndike described how behaviors followed by satisfying consequences tend to be repeated, developing sophisticated theories about the role of context and social factors in learning. Modern RL strips this to pure mechanical reward optimization, losing all nuanced understanding of social and emotional factors.
- “Federated Learning” (2017) rebranded Kropotkin’s Mutual Aid (1902). Kropotkin described how cooperation and distributed learning occur in nature and society, emphasizing knowledge development through networks of mutual support. The tech industry “discovers” distributed learning networks but focuses only on data privacy and efficiency, ignoring the social and cooperative aspects Kropotkin emphasized.
- “Explainable AI” (2016) rebranded John Dewey’s Theory of Inquiry (1938). Dewey wrote about how understanding must be socially situated and practically grounded, emphasizing that explanations must be tailored to social context and human needs. Modern XAI treats explanation as a purely technical problem, losing the rich philosophical framework for what makes something truly explainable.
- “Few-Shot Learning” (2017) rebranded Gestalt Psychology (1920s). Gestalt psychologists described how humans learn from limited examples through pattern recognition and developed sophisticated theories about how minds organize and transfer knowledge. Modern few-shot learning presents this as a novel technical challenge while ignoring deeper understanding of how minds actually organize and transfer knowledge.
These philosophical ghosts don’t just haunt our machines – they’re Wollstonecraft’s vindication made manifest, a warning echoing through centuries of wisdom. The question is whether we’ll finally listen to these voices from the margins of history, or continue pretending every thoughtless mechanical implementation of their ideas is cause to celebrate a breakthrough discovery. Remember, Caty Greene’s invention of the “cotton engine” or ‘gin (following her husband’s untimely death from over-exertion) came from intentions to abolish slavery, yet instead was stolen from her and reverse-patterned into the largest unregulated immoral expansion of slavery in world history. Today’s AI systems risk following the same pattern of automation tools intended to liberate human potential being corrupted into instruments of digital servitude.
“Naively uploading” our personal data into any platform that lacks integrated ethical design or safe learning capabilities is more like turning oneself into a slave exploited by cruelty of emergent digital factory owners, rather than maintaining basic freedoms while connecting to a truly intelligent agent that can demonstrate aligned values. Agency is the opposite to what AI companies have deceptively been hawking as their vision of agents.