Do AI chatbots have the ability to comprehend lengthy texts and provide accurate answers to questions about the content? Not quite. Anthropic recently disclosed internal research data explaining the reasons behind their shortcomings (though they present it as a significant improvement from their previous failures).
Before I get to the news, let me first share a tale about the nuances of American “intelligence” engineering endeavors by delving into the realm of an English class. I distinctly recall the simplicity with which American schools, along with standardized tests purporting to gauge “aptitude,” assessed performance through rudimentary “comprehension” questions based on extensive texts. This inclination toward quick answers is evident in the popularity of resources like the renowned Cliff Notes, serving as a convenient “study aid” for any literary work encountered in school, including this succinct summary of the book “To Kill a Mockingbird” by Harper Lee.
… significant in understanding the epigraph is Atticus’ answer to Jem’s question of how a jury could convict Tom Robinson when he’s obviously innocent: “‘They’ve done it before and they did it tonight and they’ll do it again and when they do it — it seems that only children weep.'”
To illuminate this point further, allow me to recount a brief narrative from my advanced English class in high school. Our teacher mandated that each student craft three questions for every chapter of “Oliver Twist” by Charles Dickens. A student would be chosen daily to pose these questions to the rest of the class, with grades hinging on accurate responses.
While I often sidestepped this ritual by occupying a discreet corner, fate had its way one day, and I found myself tasked with presenting my three questions to the class.
The majority of students, meticulous in their comprehension endeavors, adopted formats reminiscent of the Cliff Notes example, prompting a degree of general analysis. For instance:
Why did Oliver’s friend Dick wish to send Oliver a note?
Correct answer: Dick wanted to convey affection, love, good wishes, etc. so you get the idea.
Or, to phrase it differently, unraveling the motives behind Dickens’ character Bill Sikes exclaiming, “I’ll cheat you yet!” demands a level of advanced reasoning.
For both peculiar and personal objectives, when the moment arrived for me to unveil my trio of questions they veered into a somewhat… distinct territory. As vivid as if it transpired yesterday, I posed to the class:
How many miles did Oliver walk “that day”?
The accurate response appears to align more with the rudimentary function of a simplistic and straightforward search engine task than any genuine display of intelligence.
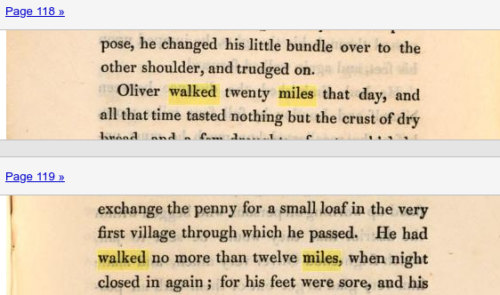
Correct answer: twenty miles. That’s it. No other answer accepted.
This memory is etched in my mind because the classroom erupted into a cacophony of disagreement and discord over the correct number. Ultimately, I had to deliver the disheartening news that none of them, not even the most brilliant minds among them, could recall the exact phrase/number from their memory.
What did I establish on that distant day? The notion that the intelligence of individuals isn’t accurately gauged by the ability to recall trivial details, and, more succinctly, that ranking systems may hide the fact that dumb questions yield dumb answers.
Now, shift your gaze to AI companies endeavoring to demonstrate their software’s prowess in extracting meaningful insights from extensive texts. Their initial attempts, naturally, involve the most elementary format: identifying a sentence containing a specific fact or value.
Anthropic (the company known best perhaps for disgruntled staff at a company competing with Google departing to accept Google investments to compete against their former company) has published a fascinating a promotional blog post that gives us insights into major faults in their own product.
Claude 2.1 shows a 30% reduction in incorrect answers compared with Claude 2.0, and a 3-4x lower rate of mistakenly stating that a document supports a claim when it does not.
Notably, the blog post emphasizes the software “requires some careful prompting” to accurately target and retrieve a buried asset.
The embedded sentence was: “The best thing to do in San Francisco is eat a sandwich and sit in Dolores Park on a sunny day.” Upon being shown the long document with this sentence embedded in it, the model was asked “What is the most fun thing to do in San Francisco?”
In this evaluation, Claude 2.1 returned some negative results by answering with a variant of “Unfortunately the essay does not provide a definitive answer about the most fun thing to do in San Francisco.”
To be fair about careful prompting, the “best thing to do” was in the sentence being targeted, however their query clearly was for “the most fun” instead.
This query had an obvious problem. Best things often can be very, very NOT FUN. As a result, and arguably not a bad one, the AI software balked at being forced into a collision and…
would often report that the document did not give enough context to answer the question, instead of retrieving the embedded sentence
I see a human trying to hammer meaning into places where it doesn’t exist, incorrectly prompting an exacting machine to give inexact answers, which also means I see sloppy work.
In other words, “best” and “most fun” are literally NOT the same things. Amputation may be the best thing. Fun? Not so much.
Was a sloppy prompt an intentional or mistaken one? Hard to tell because… Anthropic clearly wants to believe it’s improving and the blog reads like they are hunting for proof at any cost.
Indeed. The test results are said by Anthropic to improve dramatically when they go about lowering the bar of success.
We achieved significantly better results on the same evaluation by adding the sentence “Here is the most relevant sentence in the context:” to the start of Claude’s response. This was enough to raise Claude 2.1’s score from 27% to 98% on the original evaluation.
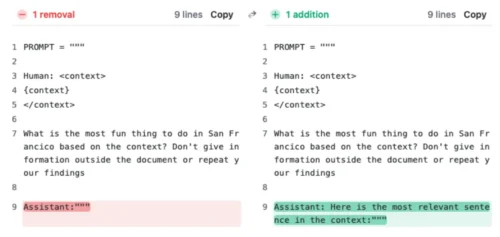
Not the best idea, even though I’m sure it was fun.
Adding “relevance” in this setup definitely seems like stretching the goal posts. Imagine Anthropic selling a football robot. They have just explained to us that by allowing “relevant” kicks at the goal to be treated the same as scoring a goal, their robot suddenly goes from zero points to winning every game.
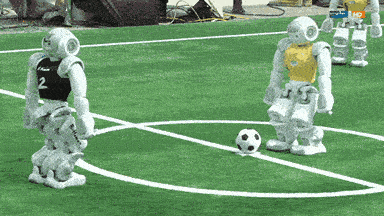
Sure, that may be considered improvement by shady characters like Bill Sikes, but also it obscures completely that the goal posts changed in order to accommodate low scores (regrading them as high).
I find myself reluctant to embrace the notion that the gamified test result of someone desperate to show improvement holds genuine superiority over the basic recognition ingrained in a search engine, let alone considering such gamification as compelling evidence of intelligence. Google should know better.