In 2014 I gave a series of talks looking at use of big data to predict effects/spread of disease, chemicals, bomb blast radius (especially in ubran areas) and how integrity controls greatly affected the future of our security industry.
This was not something I pioneered, by any stretch, as I was simply looking into the systems running on cloud by insurance companies. These companies were exhausting cloud capacity at that time to do all kinds of harm and danger predictions.
Granted I might have been the first to suggest a map of zombie movement (e.g. Russian infantry) would be interesting to plot, but the list of harm prediction goes on infinitely and everyone in the business of response wants a tool.
The 2015 electronic warfare (EW) activity in Ukraine and more recent experiences in Syria have prompted the US military to seek solutions in that area as well: given a set of features what could jamming look like and how should troops route around it, for example.
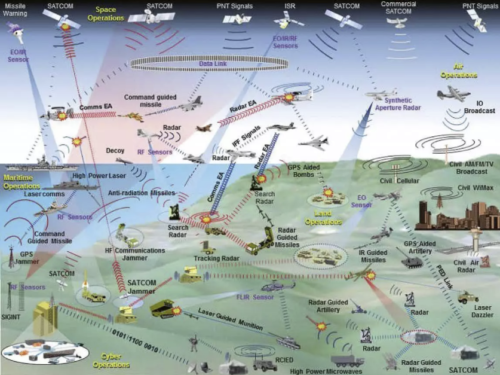
It’s a hot topic these days:
The lack of understanding of the implications of EW can have significant mission impact – even in the simplest possible scenario. For example, having an adversary monitor one’s communications or eliminate one’s ability to communicate or navigate can be catastrophic. Likewise, having an adversary know the location of friendly forces based on their electronic transmissions is highly undesirable and can put those forces at a substantial disadvantage.
The US is calling their program Electronic Warfare Planning and Management Tool (EWPMT) and contractors are claiming big data analysis development progress already:
Raytheon began work on the final batch, known as a capability drop, in September. This group will use artificial intelligence and machine learning as well as a more open architecture to allow systems to ingest swaths of sensor data and, in turn, improve situational awareness. Such automation is expected to significantly ease the job of planners.
Niraj Srivastava, product line manager for multidomain battle management at Raytheon, told reporters Oct. 4 that thus far the company has delivered several new capabilities, including the ability for managers to see real-time spectrum interference as a way to help determine what to jam as well as the ability to automate some tasks.
It starts by looking a lot like what we use for commercial wireless site assessments starting around 2005. Grab all the signals by deploying sensors (static and mobile), generate a heatmap, and dump it into a large data store.
Then it leverages commercial agile development, scalable cloud infrastructure and machine learning from 2010 onward, to generate future predictive maps with dials to modify variables like destroying/jamming a signal source.
Open architectures for big data dropping in incremental releases. It’s amazing, and a little disappointing to be honest, how 2019 is turning out to be exactly what we were talking about in 2014.